Knowledge Bot Product Recommenders Proven to Boost B2B Sales
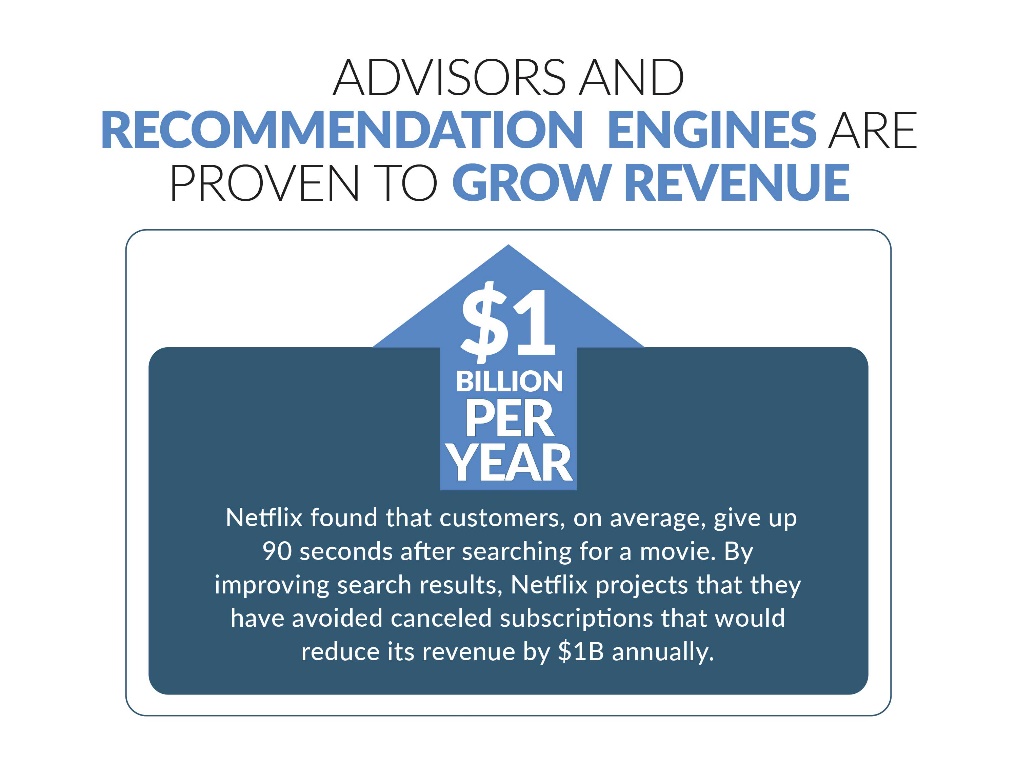
Recommendation Engines are a proven way to increase revenue in B2C. Unfortunately, B2B has not been able to capitalize on this AI-based technology because:
- B2B buyers are organizations, not a single individual
- B2B companies often purchase solutions rather than individual products
eXalt Knowledge Bots bridge this gap by offering B2B organizations a more advanced method of influencing purchase decisions and accelerating sales.
What is a Product Recommendation Engine?
The job of a Product Recommendation Engine is to recommend the most relevant items to a particular user. Buyers save the time and effort of wading through a multitude of possible products, and vendors build loyalty and drive sales through personalized experiences.
How does a Product Recommendation Engine work?
Typically, Product Recommendation Engines tailor product recommendations based on a user’s profile, past online activity and data analysis. B2C companies have been using Product Recommendation Engines for years. By moving from manual to algorithmic recommendations they have realized spectacular business success.
Product Recommendation Engines have generated eye popping growth for B2C
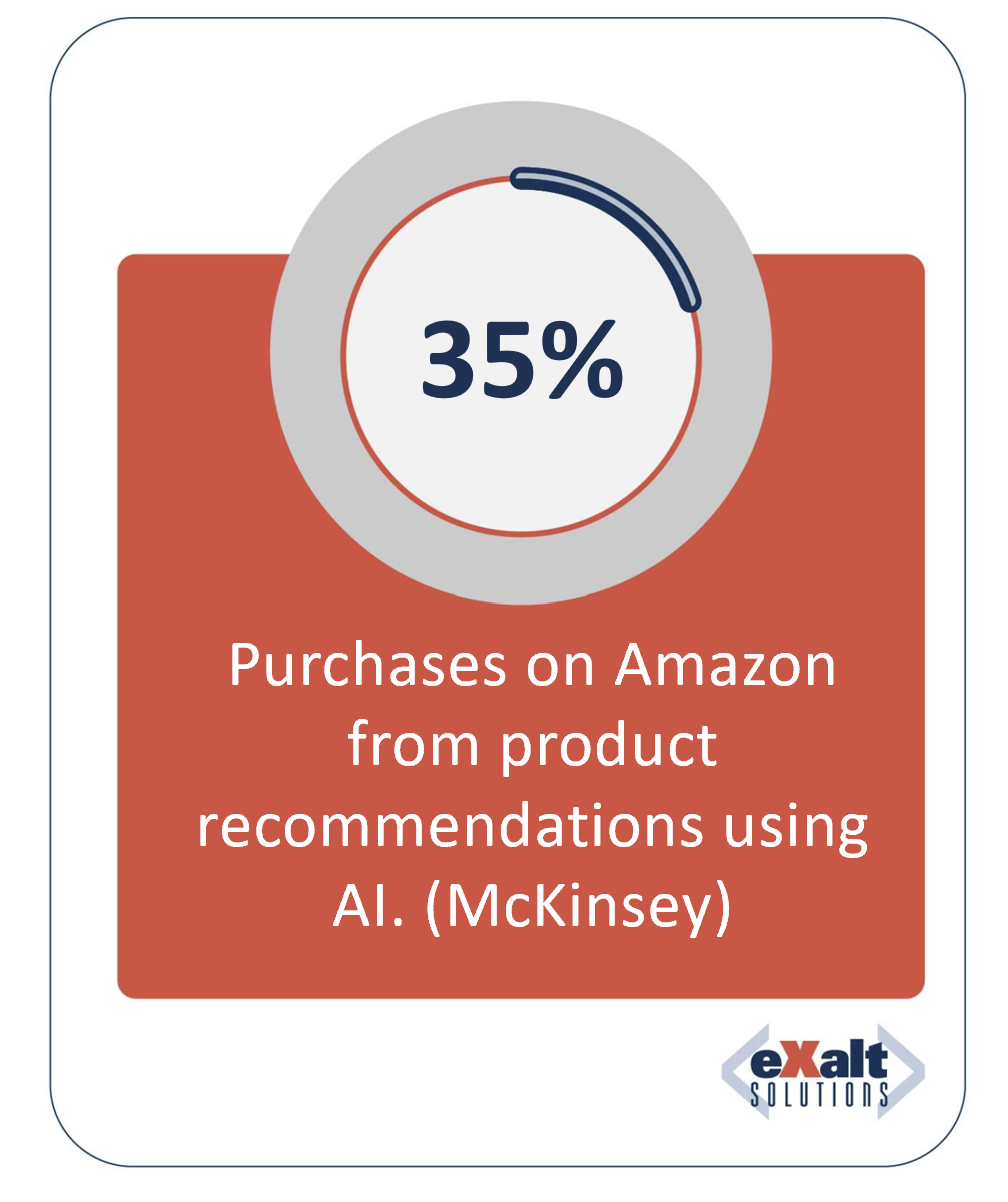
The results for B2C have been stunning:
-
35% of consumer purchases on Amazon come from product recommendations using AI. (McKinsey)
-
75% of what users watch on Netflix come from product recommendations using algorithms. (McKinsey)
-
>15% of visitors admit to buying recommended products. (Forrester)
-
9.5% more is spent by buyers who receive recommendation statements “People who viewed this also viewed” (SOURCE - Sharethis and Paley Centre for Media).
-
20% conversion rate improvement was realized by Alibaba using recommendation engines during the 11.11 Shopping Festival in 2016.
-
12% to 17% average increase in willingness to pay for a 1-star increase in recommendation rating creating. (MIT Sloan Management Review)
So why do Product Recommendation Engines work so well?
In the old days, purchases were governed by salespeople that we viewed as the “trusted advisor.” Customers asked salespeople what they recommended, or what was most popular with other customers, and made purchase decisions with this information. In the digital age, websites can employ recommendation engines in the role of “trusted advisor” by, for example, suggesting products that other people or friends have purchased or viewed.
Being empowered to make a buying decision without the pressure to buy from a salesperson is also a major advantage for time-constrained buyers.
Maybe another advantage to mention is that customers often feel overwhelmed by the number of choices of products they face—so recommendation engines help them narrow down their choices, making them more likely to purchase something (there is quite a bit of psychology research on this).
Product Recommendation Engines offer ROI that adds up quickly
The ROI from deploying Product Recommendation Engines can be measured by these key metrics:
- Engagement – by providing personalized advice that is valued by buyers your site becomes sticky.
- Retention - By providing a recommendation system and personalized user experience Netflix estimated that it has allowed them to save $1 billion per year in cancellations.
- Larger cart size – Customers are more receptive to personalized suggestions and this results in identifying potential cross-sell and up-sell opportunities that otherwise would have been overlooked by traditional methods.
- Profitability – More advanced Recommendation Engines can suggest margin making options.
Improving any of these metrics by just 10% is just a game changer for any company in any industry.
There are several types of product recommendation engines
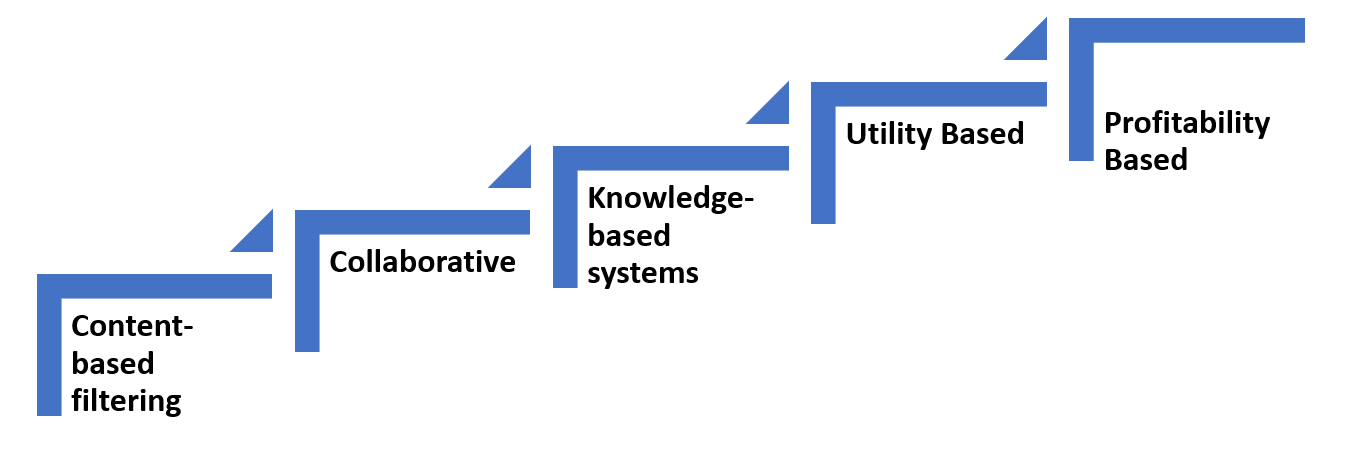
There are five (5) types of product recommendation engines:
- Content-based filtering: Recommendations are based on a single user’s interactions and preference. The browsing history of an individual who has been looking at cookbooks on Amazon is presented top selling cookbooks.
- Collaborative: Makes recommendations based on other users with similar tastes or situations. Recommendations are made based on purchase and viewing history of people with similar characteristics.
- Knowledge-based systems: Suggestions for a suitable product are based on user’s requirements and needs. These systems combine use case attributes, product attributes, solution attributes, domain expertise and knowledge with user history.
- Utility-based recommender system: This type of system makes recommendations based on a computation of its usefulness for each individual user by creating a user-specific utility function.
- Profitability-based recommender system: Instead of having recommendations solely based solely on a customer’s browsing history and past purchases, this system also ensures that the recommendation includes the most profitable options.
B2C typically uses basic Content based or Collaborative Recommendation Engines. But B2B requires more Knowledge-based recommender systems using utility or financial value.
B2B requires Advanced Knowledge Based Recommendation Engines incorporating personal utility and profitability without bias
Despite the success in B2C, B2B has not adopted Product Recommendation Engines as much due to the complexity of their businesses.
The classic content-based and collaborative Product Recommendation Engines are not well suited to B2B because:
-
In B2B companies—rather than people— are the buyers – so each purchase transaction involves several players.
-
The history of purchases is not a true indicator in most cases. You can’t say “People or companies like you have bought x,” instead you usually have to solve a business problem that is unique to the company.
-
Accordingly, you can’t just suggest a single product; you have to weave together a complete solution that solves the buyer’s business problem. Product Recommendation engines built for B2C are not suited to B2B Solutions where products are not simply selected but engineered into an overall solution
-
The AI learning for content-based and collaborative Product Recommendation engines has a major cold start issue and requires a lot of data to be collected before it is of value.
-
Often one vendor’s product recommendation is dependent upon a different vendor’s product because the products are complementary. For example, server hardware recommendations are dependent upon the software the server uses, or leasing solutions depend upon the type of capital equipment being purchased.
-
Basic Recommendation Engines do not provide the details underlying a recommendation. This lack of transparency as to why a product was suggested is insufficient in B2B where companies expect justifications for their purchases.
-
Simple Content and Collaborative Recommendation Engines can introduce unintended bias for specific products that compound over time, which means that more optimal products are under-recommended.
These limitations have prevented B2B from widely adopting Product Recommendation Engines.
If you look at most B2B websites, they focus on products, not solutions, with simple catalogs and a lot of SKUs (Stock Keeping Units). Rarely will you find an advisor to help understand the problem you are trying to solve. Instead, each buyer is left to their own devices to find SKUs one by one and combine them to create a solution. This is both time-consuming and difficult for the buyer, who may not know exactly what combination of products to buy is optimal. The major question of whether the combination of SKUs that the buyer picks is the optimal solution to their business problem is left unanswered.
Knowledge-Based Product Recommendation Systems deliver a different B2B customer experience
Advancements in artificial intelligence technology now make it possible to deliver a virtual, AI-based Advisor that can assist both buyers and sellers in crafting customized solutions to solve buyers’ problems.
eXalt’s Knowledge Bots collect user requirements for the business problem a buyer has and then assembles all the required and optional SKUs that comprise the OPTIMAL solution based on user utility for different verticals. Here are some examples:
-
DATA CENTER SERVERS: Our Knowledge Bots deliver complete solution recommendations for the data center market, including: server, memory, drive cages, drives, networking, GPUs, software applications, power supplies, and more. These Knowledge Bots optimize the solution based on a calculation of user utility, user ROI, and/or vendor profitability, so everyone wins.
-
WIRELESS NETWORKING: Our Knowledge Bots ask about the environment and space that the wireless network must operate: square footage, room shape (T, square, rectangle, etc.), indoor versus outdoor, and ambient noise. Armed with the specifications, they then determine the optimal solution from potentially hundreds of thousands of alternatives including all wireless access points, cables, software and services.
The Knowledge Bot becomes the buyer’s trusted advisor. Someone with minimal technical expertise can get a personalized answer to their problem in seconds.
Summary
Simple Recommendation Engines have generated explosive growth for B2C companies but have not been capable of addressing the more complex challenges faced by B2B and solutions sales.
But now more advanced Knowledge Based Systems are a proven way forward for B2B to deliver a different customer experience for B2B buyers. eXalt’s Knowledge Bots incorporate all the available knowledge and expertise to deliver complete recommendations based on technical characteristics, use cases, personal utility and profitability without the bias and deployment delays introduced by more simplistic systems.
Have I forgotten anything? Please let me know at lswanson@exaltsolutions.com.